The goal of any research study is to learn more about your audience by gathering accurate data that represents a population as a whole. This can only be achieved through a fair and balanced presentation of the study’s sample responses. Unfortunately, many studies are negatively impacted by various forms of bias. These biases produce distorted results and lead to incorrect conclusions. To ensure results are honest and accurate, researchers must understand what sampling bias is and how to avoid it.
What is Sampling Bias?
Sampling bias occurs when a specific portion of a population is more or less likely to be surveyed than others. Therefore, the data sample is not an accurate representation of the entire population. Sampling bias is a common issue because it may happen without the researcher’s knowledge. Sometimes a study’s design or methodology produces opportunities in the data gathering process for sampling bias to occur. This bias can appear in both probability and non-probability sampling.
Probability sampling gives each individual an equal chance for selection. It’s as though everyone’s name has been dropped into a hat. Each individual faces the same probability that their name will be drawn. However, if the list of potential participants doesn’t accurately represent the overall population, the sample could be biased. In this example case, this error would occur if someone didn’t put their name in the hat.
With non-probability sampling, participants are selected based on specific, non-random criteria. For instance, convenience sampling includes all available participants. This creates opportunities for sampling bias because people who are more accessible will be more likely to participate.
No matter the cause, sampling bias poses a real threat to research results. However, the consequences of this type of bias extend beyond skewed results. It also affects the validity of the study, gives an inaccurate understanding of relationships, and can negate the efforts of a research project, costing an organization both money and trust. Steps should be taken to avoid this bias to protect the integrity of research studies.
Different Kinds of Sampling Bias
While sampling bias can manifest in many specific ways in individual studies, they can be categorized as one of six different types of sampling bias.
1. Self-Selection
Some people have certain traits or characteristics that make them more willing participants in your study. They may even seek you out to offer to join your sample subset. As a consumer, you can see this phenomenon every day through product reviews. People who have an positive or negative emotional experience are more likely to leave a review, so the evaluation results are skewed.
2. Non-Response
Sometimes chosen participants will refuse to engage or may even drop out of a survey early. This could be due to a lack of interest, a lack of time, or they may want to avoid sharing their feelings about a topic. Either way, non-response bias can have a major impact on survey results. In a political race, if one candidate’s supporters completed an interest poll and the other candidate’s supporters didn’t, this would create a non-response bias. The results may erroneously lead the public to believe that the first candidate was ahead in the race.
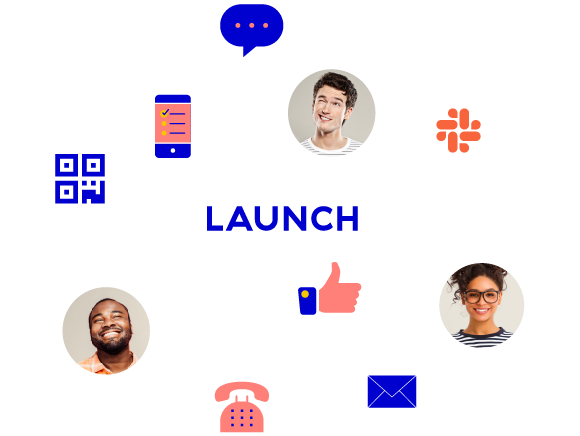
3. Undercoverage
This type of bias occurs when certain members of the overall population are under-represented in a data sample. This is a common issue with convenience sampling, as participants are selected simply because they can be reached. A dependency on easily available data may prevent researchers from revealing some truly useful information. When considering ways to reduce bias in convenience sampling, you must consider factors like demographics, social class, income level, and education. These traits can impact your research project’s resources, accessibility, and the availability of your participants.
4. Survivorship
When conducting studies, it’s easy to fall into the trap of only assessing successful people, organizations, and topics. As a result, your data reflects an overly optimistic representation of the truth. For instance, scientific journals may tend to favor research projects with positive results for their publication. This desire to omit negative data may cause some sample group members to be ignored, thus leading to skewed results.
5. Healthy User
Most often seen in medical studies, healthy user bias means that participants may be more active, healthier, and fitter than most people in the general population. By focusing on these individuals, the focus of the study shifts to equally healthy and fit people in the population. This omits people who would participate if they were healthy enough to do so from the data pool.
6. Pre-Screening or Advertising
Sometimes the pre-screening and advertising process can result in a skewed population representation. In fact, some selection criteria and advertisements that reach a highly target audience can dissuade people from participation. For instance, a study that is advertised in medical magazine commonly offered in doctor’s office waiting rooms may attract people with a heightened interest in the medical field. Consider ways to neutralize your pre-screening and advertising methods so you can reach a more realistic population sample who is interested in providing honest and reliable data.
Examples of Sampling Bias
Sampling bias can happen within any study in any field. A mental health study that asks for volunteers is more likely to receive participants who are mentally healthy. If a questionnaire is distributed to people inside a mall, the results will only represent the people who shopped in that mall on that particular day. Surveys that are written with technical jargon will discourage anyone who does not understand this jargon from partaking in the study.
For a real-life example of sampling bias, we can look at the recent global pandemic. Testing for the COVID-19 virus was a standard approach to determine the reach of the disease and help medical professionals minimize its spread. However, this sampling method tended to attract more people who were symptomatic, thus skewing the results. In response, a team with the Department of Public Health Sciences at the University of Miami Miller School of Medicine has published a new study to reduce estimation errors and correct previous sampling bias.
3 Ways to Avoid Sampling Bias
Here are three steps you can take to prevent sampling bias from occurring in your own research studies.
1. Set Clear Survey Goals
By establishing a clear understanding of what you’re trying to accomplish, you can more easily determine the most effective sample methodology and process for conducting your study. You’ll know who to contact to participate, your ideal sample size, the best way to categorize sample subsets, and how to communicate with participants for optimal results.
2. Use Random or Stratified Sampling
One effective way to avoid sampling bias is to select your study participants at random. This way, every individual has an equal chance of being included in the sample group. Some options include choosing names from a list, picking a number from a hat, or spining a wheel to land on a color. Whatever you do, make sure it’s completely random.
You might also consider stratified sampling, which takes the overall population and creates a proportionate subset of samples. For instance, if your total population consists of 1,000 people (500 men and 500 women) and your study only needs 10 participants, your sample group should include five men and five women. This maintains the same proportion as the whole population, helps regulate samples, and protects your study from sampling bias.
3. Ask the Right Questions
It’s easy to word questions in a way that limits the responder’s answers. Don’t ask participants to select answers from a list. You could fail to include every available option, which would skew responses and results. Instead, use open-ended questions that encourage people to leave their own honest answers. This enables you to gain a more complete perspective and generates more helpful data.
Avoid Sampling Bias with CheckMarket
At CheckMarket, we know how important sample selection is for your research. Our easy-to-use online survey software helps businesses identify an effective sample subset and gain insight that’s representative of the larger population. Let us help you build the samples you need and gather valuable data that can shape your overall business strategies.
Geef een antwoord